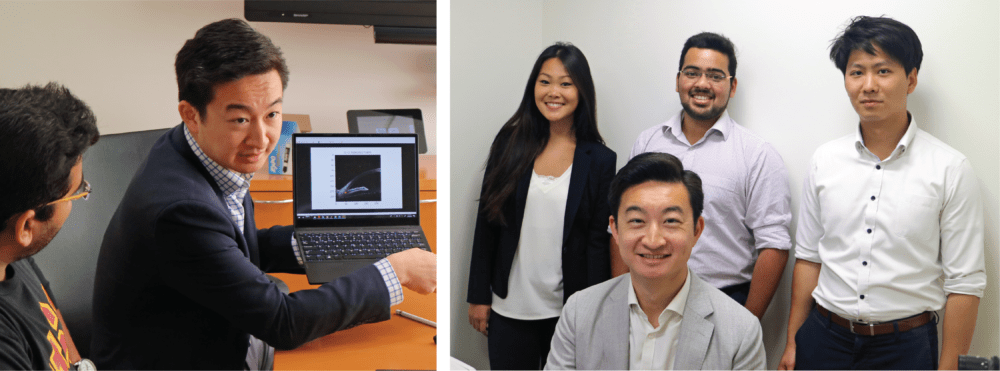
Research Overview
Primary angle closure glaucoma (PACG) is a leading cause of permanent vision loss worldwide, affecting an estimated 16 million people. PACG is the most severe stage of primary angle closure disease (PACD), which also includes primary angle closure suspect (PACS) and primary angle closure (PAC). The primary risk factor for developing PACG is closure of the anterior chamber angle (ACA), which leads to impaired aqueous humor outflow and elevated intraocular pressure (IOP).
Early and accurate identification of individuals with angle closure is crucial to prevent PACG-related vision loss. Gonioscopy, the current clinical standard for evaluating the ACA and detecting angle closure, has several limitations (Figure 1). Gonioscopy is subjective, qualitative, and requires a trained examiner with considerable expertise.

Anterior segment optical coherence tomography (AS-OCT) is a non-contact imaging method that produces qualitative images and quantitative measurements of anterior segment structures, including the ACA (Figure 2). AS-OCT imaging can be performed by a technician with minimal training. However, the adoption of AS-OCT by clinicians has been slow due to a lack of methods to support its clinical utility. The Xu lab focuses on developing novel clinical and research methods to increase the utility of AS-OCT and improve the care of patients at risk for PACG.
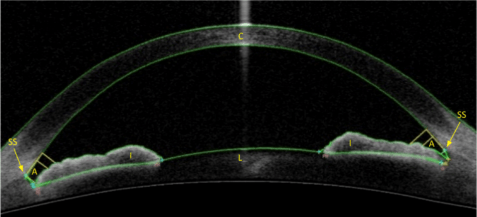
Research Topics
- Development and validation of novel OCT-based methods to assess patient risk for Primary Angle Closure Glaucoma (PACG)
- Artificial intelligence (AI) and development of automated methods to detect risk factors for PACG
- Epidemiology and identification of risk factors for Primary Angle Closure Disease (PACD)
- Characterization of physiological and biomechanical properties of intraocular structures and aqueous outflow pathways
Ongoing Projects
Project 1: Development and Validation of Novel OCT-based Methods to Assess Patient Risk for Primary Angle Closure Glaucoma (PACG)Project 2: Artificial intelligence (AI) and Development of Automated Methods to Detect Risk Factors for PACGProject 3: Epidemiology and Risk Factors of Primary Angle Closure Disease (PACD)Project 4: Characterization of Physiological and Biomechanical Properties of Intraocular Structures and Aqueous Outflow Pathways
Project 1: Development and Validation of Novel OCT-based Methods to Assess Patient Risk for Primary Angle Closure Glaucoma (PACG)
The primary focus of the Xu lab is to develop novel clinical and research methods to evaluate and manage patients with angle closure. In one aspect of this work, we study the relationship between gonioscopy and AS-OCT to better understand the strengths and weakness of each assessment method. We also seek to demonstrate and validate the utility of quantitative AS-OCT measurements. For example, we demonstrated that AS-OCT measurements of ACA width are related to IOP, an important risk factor for glaucoma (Figure 3). Therefore, AS-OCT may play an increasingly important role in the clinical evaluation and management of patients with angle closure.
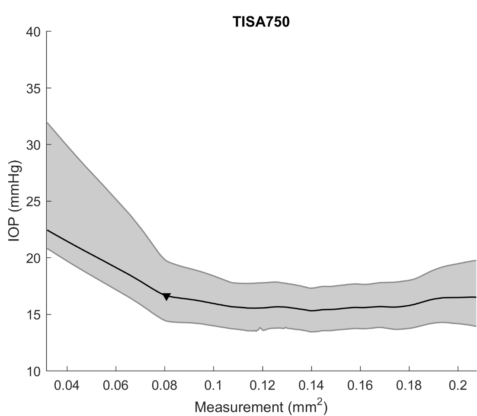
Project 2: Artificial intelligence (AI) and Development of Automated Methods to Detect Risk Factors for PACG
Artificial intelligence (AI) and specifically deep learning has gained popularity as a method for analyzing imaging data to automate the diagnosis of disease. Therefore, deep learning is a logical approach to develop automated methods to detect patients at risk for PACG. For example, the Xu lab has developed algorithms to automate the analysis of AS-OCT images for detecting gonioscopic angle closure (Figure 4). The need for these types of automated methods is especially urgent since the prevalence of PACG is highest in parts of the world with limited access to eyecare.

Project 3: Epidemiology and Risk Factors of Primary Angle Closure Disease (PACD)
The Xu lab applies biostatistical tools, including machine learning, to perform large volume analysis of databases from population-based epidemiological studies. This includes data from the Chinese American Eye Study (CHES), an NIH-funded epidemiological study of Chinese Americans in Los Angeles, a population at high risk for PACG (Figure 5). This work, performed in collaboration with members of the USC Department of Preventive Medicine, seeks to advance our understanding of the prevalence of PACD in the United States and identify novel risk factors for PACG.
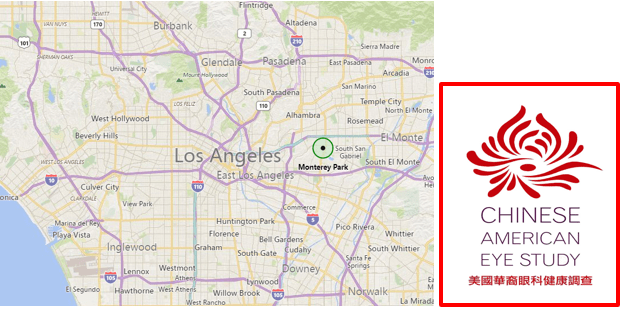
Project 4: Characterization of Physiological and Biomechanical Properties of Intraocular Structures and Aqueous Outflow Pathways
The Xu lab collaborates with faculty in the USC Viterbi Department of Biomedical Engineering to study the biological properties of intraocular structures that contribute to the pathogenesis of PACD, such as the iris. We work with our collaborators to develop novel experimental devices and software algorithms to study the dynamic behavior of intraocular structures in normal and angle closure eyes.
Video 1: An example of custom hardware and software designed to study the dynamic behavior of the pupil and iris.